What are the Big data driving factors
Big data mastering is more like a trip, not a one-time project. It is the nature of the beast which dominates every epistemological effort by "noise over signal." If creativity is to be pursued a certain amount of project failure should be accepted. Taking all the key factors listed above into consideration, organizations can make progress in driving Big Data performance. The topic is in trend, but Big Data projects have a very low success rate, so the real question is how organizations cope with the situation.To learn complete course visit ITGuru big data training Blog
In this article let us discuss the driving factors of Big data.
1. Have a Market Target in Mind
It needs to be clear what the outcomes of the project will be, who can or will benefit and how they can benefit. It is essential that you have the right team in place at the end of the day to make use of the insights that come from the projects, what better decision. You'll be able to make those better decisions as a result of the analysis and how you drive and track them. It is understandable that you have started many BDA projects. But have no established business driver or necessity in a competitive internal
environment. So most of these projects do not work.
The failures will continue to pile up as long as Big Data projects tend to be seen as pure IT projects. The problem usually isn't keeping the machines going, it's the inability to generate value because end-users
and key decision makers are ill prepared to "get it". Then extract and use the inherent value at the disposal of the mass of the data. The business purpose of the Big Data project is to convert big data into
understandable, digestible, useful and actionable information.
Data imperfection and Immature technology
In Big Data Analytics, data will be incomplete and less than perfect (duplicates, incompleteness, etc.) and the software will have to handle the actual state of the data. By the time a project begins, there is
typically about 30 percent more data available both in quantity and diversity.The analytics need to be improved and adjusted, because when you start, you don't know anything. Your analytics development
and deployment framework should encourage an iterative development approach
The industry is plagued by lack of resources, high volume of data, new technology that isn't ready for production, variety of data sources and high volume of data. This is also promoting innovation at a very
fast rate, but it will take time for technology to evolve and stabilize, and then the success rate will increase, more in line with, or even higher, industry-standard project success rate.
3. Talented professionals in big dataThe stumbling block of most of these projects is because Big Data seems like a disruptive technology and the system 's old users are not equipped with these new concepts and will go a long way to see it fail.
In this article let us discuss the driving factors of Big data.
1. Have a Market Target in Mind
It needs to be clear what the outcomes of the project will be, who can or will benefit and how they can benefit. It is essential that you have the right team in place at the end of the day to make use of the insights that come from the projects, what better decision. You'll be able to make those better decisions as a result of the analysis and how you drive and track them. It is understandable that you have started many BDA projects. But have no established business driver or necessity in a competitive internal
environment. So most of these projects do not work.
The failures will continue to pile up as long as Big Data projects tend to be seen as pure IT projects. The problem usually isn't keeping the machines going, it's the inability to generate value because end-users
and key decision makers are ill prepared to "get it". Then extract and use the inherent value at the disposal of the mass of the data. The business purpose of the Big Data project is to convert big data into
understandable, digestible, useful and actionable information.
Data imperfection and Immature technology
In Big Data Analytics, data will be incomplete and less than perfect (duplicates, incompleteness, etc.) and the software will have to handle the actual state of the data. By the time a project begins, there is
typically about 30 percent more data available both in quantity and diversity.The analytics need to be improved and adjusted, because when you start, you don't know anything. Your analytics development
and deployment framework should encourage an iterative development approach
The industry is plagued by lack of resources, high volume of data, new technology that isn't ready for production, variety of data sources and high volume of data. This is also promoting innovation at a very
fast rate, but it will take time for technology to evolve and stabilize, and then the success rate will increase, more in line with, or even higher, industry-standard project success rate.
3. Talented professionals in big dataThe stumbling block of most of these projects is because Big Data seems like a disruptive technology and the system 's old users are not equipped with these new concepts and will go a long way to see it fail.
There are usually two distinct skill sets, technical on the architectural side and the collection of analytical skills to produce information based practice. Both of you need a solid team to get a shot. If not, you've wasted time , money, effort and potentially damaged prospects for coming down the pike on the next projects. For instance, if you have a new project that is capable of connecting your CRM and other support systems into a nice wrapper and you can see how sales, the loyalty program and your customer service activities can help someone place their customers in groups, it doesn't do any good if there's no one in marketing or in a specialized analytical role or group that can do something with that knowledge, like understanding it.
The statistician is no longer a magician and you must not expect to produce gold from any useless substance given to him. He is more like a chemist able to measure precisely how much value he contains, and also capable of extracting that quantity, and no longer. In these conditions, it would be
irrational to applaud a statistician because his results are accurate or to reprove because they are not.
4. Collaborate with Right Talent Processes
Weak planning and teamwork are related to organization and project management, but the essence of most big data projects requires the ability to read and process data varieties quickly, high volume data sources, making coordination crucial. As the nature of these data sources and the business needs continue to evolve, things get complicated very quickly. If companies are unable to employ the right type of data scientist(s) with all the expertise to advance the project and understand the business needs, they will end up hiring a lot of individuals to fulfill the skill set needs. This creates a problem of
communication and coordination … through contact touch points with the square number of people involved in the project. And we know how things go.
That has remained constant, or at least linearly increased, is the number of prospective data users who seek magic over statistics, equate computational speed with statistical precision, and generally prefer the answers they seek over the answers given to them by past analysis, regardless of scale.
On the business side, companies have still not fully understood how business processes can be updated so they can take advantage of what big data can theoretically say. Weak coordination, poor planning and lack of skills should however be classified as organizational failures rather than project failures
based on Big Data.
5. Managing the Big Data Process
The statistician is no longer a magician and you must not expect to produce gold from any useless substance given to him. He is more like a chemist able to measure precisely how much value he contains, and also capable of extracting that quantity, and no longer. In these conditions, it would be
irrational to applaud a statistician because his results are accurate or to reprove because they are not.
4. Collaborate with Right Talent Processes
Weak planning and teamwork are related to organization and project management, but the essence of most big data projects requires the ability to read and process data varieties quickly, high volume data sources, making coordination crucial. As the nature of these data sources and the business needs continue to evolve, things get complicated very quickly. If companies are unable to employ the right type of data scientist(s) with all the expertise to advance the project and understand the business needs, they will end up hiring a lot of individuals to fulfill the skill set needs. This creates a problem of
communication and coordination … through contact touch points with the square number of people involved in the project. And we know how things go.
That has remained constant, or at least linearly increased, is the number of prospective data users who seek magic over statistics, equate computational speed with statistical precision, and generally prefer the answers they seek over the answers given to them by past analysis, regardless of scale.
On the business side, companies have still not fully understood how business processes can be updated so they can take advantage of what big data can theoretically say. Weak coordination, poor planning and lack of skills should however be classified as organizational failures rather than project failures
based on Big Data.
5. Managing the Big Data Process
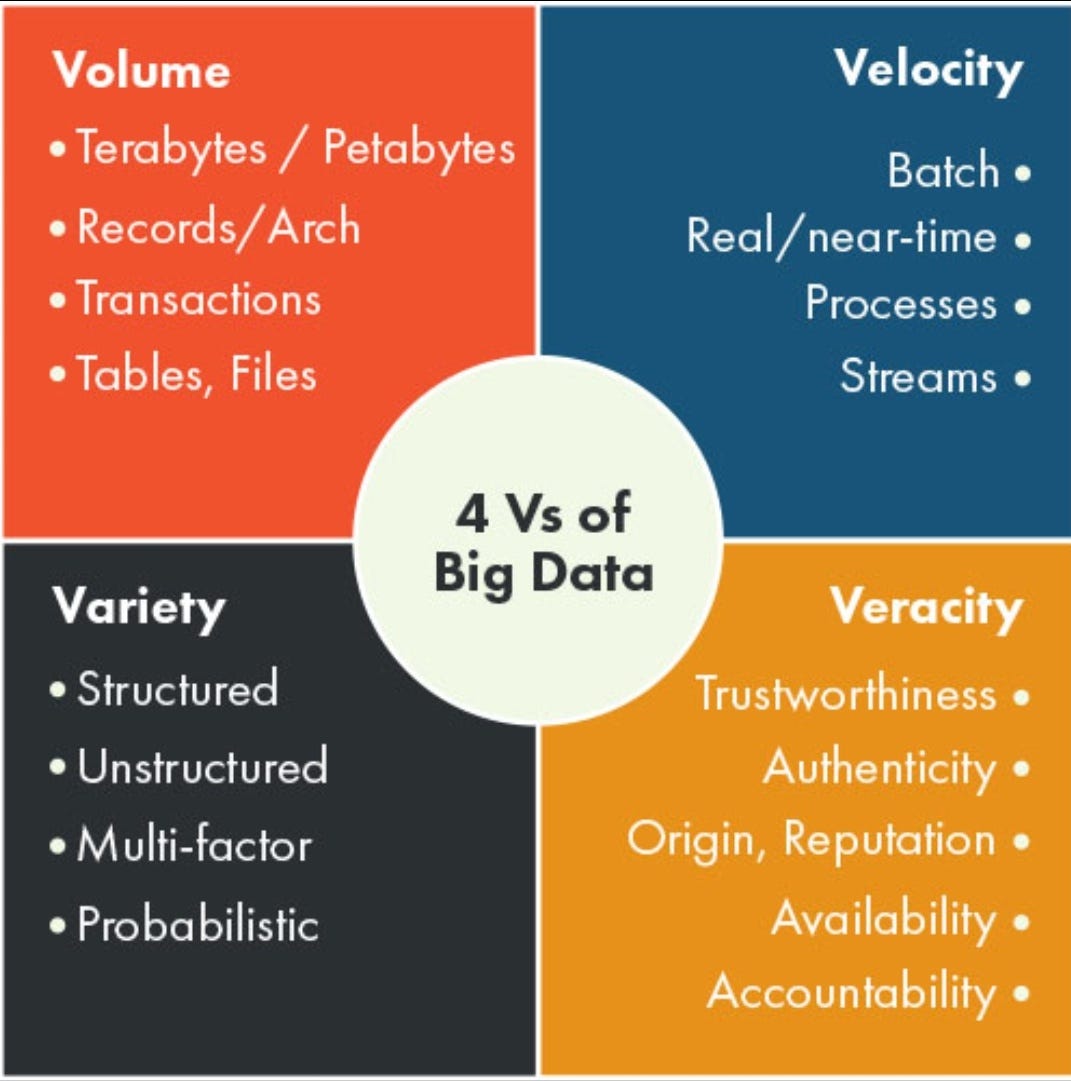
Big data's life cycle is to assess capacity planning for Big data services over timely intervals and planned growth cycles. In the collection processes, the velocity and a metric for relevant time sensitivity is important for what you are analyzing and for what purpose, according to the predictive analytics
needed to support the model coupled with levels of complexity.
For several decades of data management, IT has been moving towards a "single source of truth." There's a lot of money and technology going to get everything to line up and agree. There is a whole "Master Data Management" industry that has evolved out of the desire for consistency and correctness- regardless of the legal requirements to get the "right" data. Big data often threatens all of this and
makes many IT shops nervous, so projects get sidetracked, slowed down or abandoned as it takes the new angle and multi-dimensional lens to capture insight into big data.
Until organizations have an operational context that can react to new capabilities, the project can be technically successful, but never provide sufficient business value to support them. You probably won't
get to do another one in that case …. Big Data has all the tough features, it's necessary to think through how to build the Big Data modeling framework as a systematic approach to master volume , speed and
complexity.
Conclusion:
I hope you reach a conclusion about the driving factors of Big Data. I hope you are driven by these factors. If you want to learn Big Data you can go for big data online training.
needed to support the model coupled with levels of complexity.
For several decades of data management, IT has been moving towards a "single source of truth." There's a lot of money and technology going to get everything to line up and agree. There is a whole "Master Data Management" industry that has evolved out of the desire for consistency and correctness- regardless of the legal requirements to get the "right" data. Big data often threatens all of this and
makes many IT shops nervous, so projects get sidetracked, slowed down or abandoned as it takes the new angle and multi-dimensional lens to capture insight into big data.
Until organizations have an operational context that can react to new capabilities, the project can be technically successful, but never provide sufficient business value to support them. You probably won't
get to do another one in that case …. Big Data has all the tough features, it's necessary to think through how to build the Big Data modeling framework as a systematic approach to master volume , speed and
complexity.
Conclusion:
I hope you reach a conclusion about the driving factors of Big Data. I hope you are driven by these factors. If you want to learn Big Data you can go for big data online training.
No comments: